Construction and validation of a prognostic signature for mucinous colonic adenocarcinoma based on N7-methylguanosine-related long non-coding RNAs
Highlight box
Key findings
• The N7-methylguanosine (m7G)-related long non-coding RNA (lncRNA) prognostic signature has potential value for the prognosis and diagnosis of mucinous colonic adenocarcinoma.
What is known and what is new?
• Mucinous colonic adenocarcinoma is characterized by its propensity to metastasize and recur, resulting in a poor prognosis.
• Our study suggested that m7G-lncRNA related prognostic signature may be a valuable biomarker for the diagnosis and treatment for mucinous colonic adenocarcinoma.
What is the implication, and what should change now?
• The development of a novel diagnostic and prognostic signature may help develop new disease prevention measures and help improve patient prognosis.
Introduction
Colorectal cancer (CRC) is one of the most common digestive system tumors, accounting for about 10.0% of all cancers worldwide (1). Recently, mortality from CRC has declined, but the 5-year survival rate for patients with metastatic CRC is still less than 20% (2). CRC can metastasize to multiple organs or tissues, posing a major threat to human health. According to the World Health Organization classification criteria, CRC can be divided into three subtypes: non-mucinous adenocarcinoma (NMC), mucinous adenocarcinoma (MC), and signet-ring cell carcinoma (SRCC). MC is the second most common pathological type and accounts for about 10–15% of CRC cases (3). Compared with that of patients with the NMC subtype, the survival rate of those with MC is consistently lower. Additionally, 50% of MC tissues are composed of extracellular mucinous proteins, with high microsatellite instability and a poor response to systemic treatment (4). Therefore, it is necessary to develop a prognostic signature of mucinous colonic adenocarcinoma for improved diagnosis and prognosis.
Long non-coding RNA (lncRNA) is a type of RNA with a length of more than 200 bp but no protein-coding function (5). LncRNAs are widely distributed in the cytoplasm and nucleus, figuring prominently in gene regulation (6,7). In recent years, it has been found that the lncRNA can act as cis- or trans-factors at the transcriptional, post-transcriptional, or translational levels, which may contribute to the occurrence and development of cancer (8). LncRNAs have been proposed as biomarkers for cancer. For instance, lncRNA has been demonstrated to be a serum diagnostic biomarker for the diagnosis of cervical cancer (9). In addition, the level of lncRNA-p21 was shown to be significantly increased in prostate cancer and thus may be used as a biomarker for the diagnosis of prostate cancer (10). A study has reported that the expression of lncRNA TP53 TG1 is downregulated in gastric cancer, functioning as a tumor suppressor (11). Identifying the differential expression of lncRNAs in tumors plays a role in promoting tumorigenesis and tumor suppression, providing opportunities for the development of new cancer therapies based on targeting lncRNAs.
RNA modification is an important component of post-transcriptional regulation and occurs in almost all types of RNA. More than 170 types of RNA modifications have been identified, which are involved in regulating various biological functions (12). N7-methylguanosine (m7G), a modification type present at the 5’cap of RNA and internal messenger RNA, is one of the most heavily methylated modifications (13). m7G is achieved by the methyltransferase METTL1/WDR4 complex, which catalyzes the addition of methyl groups to the 7th N position of guanosine (G) in RNA. m7G affects various physiological and pathological processes by regulating RNA metabolism (14). A variety of studies have shown that m7G METTL1 or WDR4 is involved in regulating the occurrence and development of various cancers, such as liver cancer (15), head and neck squamous cell carcinoma (16), bladder cancer (17), colon cancer (18) and so on. In addition, m7G-related genes have been used to construct a prognostic model of the liver (19).
At present, several articles have used bioinformatics analysis to explore the relationship between m7G-associated lncRNAs and colon cancer by constructing different models to predict effective biomarkers (20-23). However, the above studies mainly focus on colon cancer and colon adenocarcinoma (COAD), with wider range of prognostic model predicts, and the accuracy of the model needs to be improved. In addition, the role of m7G modification-related lncRNAs in the progression of mucinous colonic adenocarcinoma remains uncertain. Therefore, finding m7G-related lncRNA biomarkers is crucial for early identification and prognostic evaluation of mucinous colonic adenocarcinoma.
Hence, based on the MC patient data obtained from The Cancer Genome Atlas (TCGA) dataset, as well as bioinformatics and statistical analyses, we created an m7G-related lncRNA prognostic signature to reliably predict the survival status of MC patients. Additionally, we discussed the clinical value, tumor immune cell invasion, and predictive value of tumor mutational burden (TMB) of related lncRNAs in MC. Our study provides further insight into the prognosis for MC of CRC. The flowchart in Figure 1 shows the process of data collection, data analysis, and data visualization in our study. We present this article in accordance with the TRIPOD reporting checklist (available at https://jgo.amegroups.com/article/view/10.21037/jgo-23-980/rc).
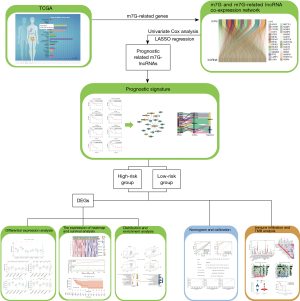
Methods
Data set
We extracted transcriptomic data and clinical information of 113 patients from TCGA database (https://cancergenome.nih.gov/), including 41 cases of adjacent tissues and 72 cases of MC tissues of colon. The raw read counts in the transcriptome data were voom normalized via the “limma” package (24) in R software (The R Foundation of Statistical Computing, Vienna, Austria). Table 1 summarizes the clinicopathological characteristics of the patients. Patients without clinical information were excluded from the subsequent analysis. A total of 39 m7G-related regulators (Table S1) were obtained from the Gene Set Enrichment Analysis (GSEA) website (https://www.gsea-msigdb.org). The study was conducted in accordance with the Declaration of Helsinki (as revised in 2013).
Table 1
Variables | Value (n=72) |
---|---|
Age (years), n (%) | |
≤65 | 26 (36.1) |
>65 | 38 (52.8) |
Unknown | 8 (11.1) |
Gender, n (%) | |
Female | 32 (44.4) |
Male | 32 (44.4) |
Unknown | 8 (11.1) |
Pathological stage, n (%) | |
I | 10 (13.9) |
II | 26 (36.1) |
III | 21 (29.2) |
IV | 7 (9.7) |
Unknown | 8 (11.1) |
T stage, n (%) | |
T1 | 1 (1.4) |
T2 | 9 (12.5) |
T3 | 42 (58.3) |
T4 | 12 (16.7) |
Unknown | 8 (11.1) |
N stage, n (%) | |
N0 | 37 (51.4) |
N1 | 14 (19.4) |
N2 | 13 (18.1) |
Unknown | 8 (11.1) |
M stage, n (%) | |
M0 | 46 (63.9) |
M1 | 7 (9.7) |
MX | 10 (13.9) |
Unknown | 9 (12.5) |
MC, mucinous adenocarcinoma.
Selection of m7G-related lncRNAs
We performed Pearson correlation analysis using the “limma” package in R and identified 432 lncRNAs (Table S2) associated with m7G. The Pearson analysis criteria used were |Pearson correlation coefficient| >0.4 and P value <0.001. R packages including “ggplot2” (25), “ggalluvial” (26), and “dplyr” were used to visualize the correlation results as Sankey diagrams.
Further screening for the prognosis-associated m7G-related lncRNAs
Univariate Cox regression analysis was used to select lncRNAs associated with m7G and with prognostic significance (P<0.01). To further screen and establish a prognostic signature, least absolute shrinkage and selection operator (LASSO) regression analysis was performed. According to the results of LASSO regression analysis, the survival analysis of the selected related genes was carried out. The R software packages “survival” and “glmnet” (27) and Cytoscape 3.8 software were used to generate forest plots, a LASSO regression model diagram, a co-expression network figure, and Sankey diagrams.
Construction and validation of the m7G-related lncRNA prognostic signature and nomogram
We used the corresponding regression coefficients of m7G-related lncRNAs and their expression levels to construct a feature called risk score. The risk score model for each patient was calculated as follows:
where Coefi and xi are regression coefficients and expression levels of each m7G-related lncRNA, respectively. Subsequently, patients were divided into low- and high-risk groups based on the median risk score of the above-described model, and randomly assigned to the training set and the test set with a ratio of 6:4. Heatmaps, scatter plots, and Kaplan-Meier survival curves were drawn to evaluate the difference in overall survival (OS) between the two subgroups. In addition, receiver operating characteristic (ROC) curves, and area under the ROC curves (AUCs) were constructed to determine the predictive accuracy of the prognostic signature. Finally, based on the independent prognostic factors (risk score) selected from the univariate and multivariate regression analysis, the corresponding nomogram was drawn to evaluate the validity of the signature, and the accuracy of the nomogram was evaluated with a calibration graph. The “pheatmap”, “xfun”, “survival”, “survminer”, and “timeROC” R packages (28) were used to draw the above-mentioned graphs.
Principal component analysis (PCA) and functional enrichment analysis
To determine the distribution of patients with different risk scores, PCA was performed using the R software package “scatterplot3D”. Subsequently, the R “limma” package was used to analyses the difference in expression levels of different risk groups, and the Kyoto Encyclopedia of Genes and Genomes (KEGG) and Gene Ontology (GO) KEGG Orthology-Based Annotation System (KOBAS) gene annotation tool (http://kobas.cbi.pku.edu.cn/genelist/) was used for pathway enrichment of genes with significant differences. Then the results of KEGG and GO enrichment were visualized using the R package “readr”. When |log2fold change| >1 and P value <0.05, these genes were considered to be significantly different.
Analysis of immune cell invasion
Based on the above risk model, the risk population has been divided into two subgroups: low- and high-risk. We obtained the LM22 gene set from the CIBERSORT website (http://cibersort.stanford.edu/) to estimate the total immune infiltration in each MC sample and immune cell subsets. The CIBERSORT algorithm was used to score 21 immune cells, and a matrix of 1,000 permutations was used to calculate the CIBERSORT P values. The “vioplot” and “corrplot” R software packages were used to visualize the differential expression of immune cell infiltration (ICI) in the different risk groups and the correlation between different immune cells. Finally, Spearman rank correlation coefficient was used to evaluate the correlation between different tumor immune cell types. The threshold for screening different risk groups was set to P<0.05.
Analysis of TMB
Somatic mutation data of mucinous colonic adenocarcinoma was obtained from TCGA database, and the TMB of each sample were calculated. The TMB differences between the different risk groups were visualized, and Kaplan-Meier curves were plotted for the low- and high-risk groups. The results were visualized using the “maftools” (29), “limma”, “ggpubr”, and “survival” R software packages.
Analysis of immunophenotype score (IPS)
IPS determines immunogenicity by referring to effector cells, immunosuppressive cells, MHC molecules, and immunomodulators. The IPS results of TCGA-COAD patients were downloaded from The Cancer Immunome Atlas (TCIA) (https://tcia.at/home). We visualized the IPS analysis results using “reshape2”, “ggpubr” R packages.
m7G-related lncRNAs modification prediction
In theory, direct RNA sequencing can detect any given modification in a natural RNA molecule in real-time and simultaneously (30). We used the m7GFinder predictor in the m7GHub V2.0 database to predict whether the relevant lncRNAs are likely to undergo m7G modification (31,32).
Statistical analysis
All analyses in this study were performed using R software (version 4.1.0). Unless otherwise noted, statistical significance was set at P<0.05.
Results
Identification of m7G-related lncRNAs and construction of the prognostic signature
In TCGA-COAD dataset, we selected 72 samples of MC tissue and 41 adjacent tissues. To investigate the association between m7G and MC, we obtained a set of 39 genes identified as regulators of m7G from the GSEA website. The expression levels of these genes and lncRNAs were used in a co-expression analysis, resulting in the identification of 432 lncRNAs associated with m7G (Figure 2A).
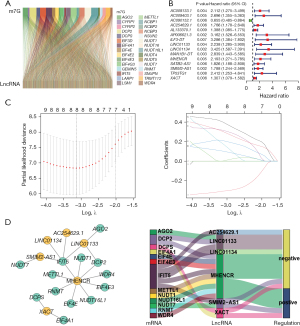
Subsequently, univariate Cox regression analysis was performed to identify 15 m7G-related lncRNAs (P<0.01): AC009133.1, AC009403.1, AC090152.1, AC254629.1, AL133370.1, AP006621.3, ILF3-DT, LINC01133, LINC01134, MAN1B1-DT, MHENCR, SATB2-AS1, SMIM2-AS1, TP53TG1, and XACT (Figure 2B). To further screen for prognostic factors, LASSO regression analysis was performed on these 15 genes. We determined the optimal parameter λ via 1000-fold cross-validation and calculated the corresponding coefficients based on the minimum corresponding criterion (Table 2) to select eight genes: AC090152.1, AC254629.1, LINC01133, LINC01134, MAN1B1-DT, MHENCR, SMIM2-AS1, and XACT (Figure 2C).
Table 2
LncRNA | Coefficient |
---|---|
AC090152.1 | −0.308122075740802 |
AC254629.1 | 0.000526087922221737 |
LINC01133 | 0.211606769910181 |
LINC01134 | 0.182367469016534 |
MAN1B1-DT | 0.317633432140595 |
MHENCR | 0.403254381313619 |
SMIM2-AS1 | 0.306374489757572 |
XACT | 0.103634371005555 |
m7G, N7-methylguanosine; lncRNA, long non-coding RNA.
Finally, Kaplan-Meier survival analysis was performed on these eight lncRNAs, and the results showed that the low expression of six genes was associated with good prognosis (Figure S1). The subsequent analysis was based on these six genes. The interaction network between m7G-related lncRNAs and m7G regulators (Figure 2D) consisted of six lncRNAs and 13 regulators. The correlation of the six lncRNAs with the target genes was visualized by Sankey diagram and included positive and negative correlations (Figure 2D).
Clinical significance of the m7G-related lncRNA prognostic signature
To verify the clinical significance of these lncRNAs, differential expression analysis was performed. As shown in Figure 3A, only the expression of MHENCR in cancer tissues was slightly higher than that in adjacent tissues, and the expression of the other genes in cancer tissues was significantly lower than that in adjacent tissues. We then also examined the relationship between the expression of m7G-related lncRNAs and clinicopathological features. The results showed that the expression of six prognosis-related lncRNAs was significantly different in the different molecular subtypes of colon cancer (P<0.001) (Figure 3B). In addition, the expression of XACT was significantly different in different stages (stages I, II, III, and IV) and also varied according to T stage (T1, T2, T3, and T4), while the remaining genes showed no significant difference in these pathological stages (Figure 3B).
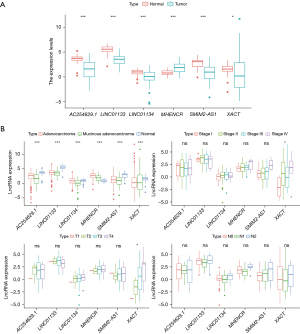
Validity of the m7G-related lncRNA prognostic signature
Based on the risk scoring model described above, we divided the patients into two subgroups: low- and high-risk. The heat maps of both the training set and the test set showed different expression patterns of six lncRNAs between high-risk and low-risk groups (Figure S2A,S2B), and the entire set yielded similar results (Figure 4A). The hazard curves and scatter plots in Figure 4B show that patients with higher risk scores had worse survival, as well as results in the training set and test set (Figure S2C,S2D). We also performed a Kaplan-Meier analysis to predict survival, which showed that the low-risk group had a favorable prognosis (Figure 4C, Figure S2E,S2F). Moreover, the AUC values for 3-, 5-, and 10-year survival of the training set were 0.944, 0.957, and 1.000, respectively (Figure S2G). And in the test set were 0.964, 1.000, and 1.000, respectively (Figure S2H). These results indicated that the m7G-lnRNAs signature could predict prognosis for patients of MC.
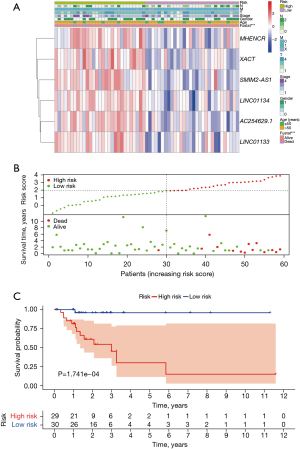
Subsequently, univariate and multivariate Cox regression and ROC analysis were performed to determine whether clinicopathological characteristics could serve as reliable prognostic factors for MC. Univariate and multivariate regression analyses of clinical characteristics and risk score were performed for patients with MC. The results of risk score were [hazard ratio (HR): 6.458, 95% confidence interval (CI): 2.708–15.403, P<0.001; HR: 7.280, 95% CI: 2.500–21.203, P<0.001], respectively (Table 3). Thus, the risk score could be considered an independent prognostic factor for MC. Next, we constructed ROC curves for age, sex, pathological stage, and risk score. As shown in Figure 5A, risk score had an AUC of 0.961, which was significantly higher than those of the other clinical variables. The AUC values of the risk score as an independent prognostic factor for 3, 5, and 10 years were 0.911, 0.955, and 0.961, respectively. Finally, we included age and risk score in the nomogram (Figure 5B) and performed nomogram calibration. The results showed that the calibration model and ideal line were very close, indicating good calibration (Figure 5C). These results provided further validation of the reliability of the risk score as a prognostic factor for MC.
Table 3
Characteristics | Univariate analysis | Multivariate analysis | |||
---|---|---|---|---|---|
HR (95% CI) | P value | HR (95% CI) | P value | ||
Age (years) | 1.008 (0.996–1.051) | 0.720 | 1.066 (1.005–1.130) | 0.033 | |
Gender | 1.025 (0.358–2.935) | 0.963 | 0.545 (0.119–2.491) | 0.434 | |
Stage | 1.915 (0.992–3.696) | 0.053 | 0.623 (0.140–2.769) | 0.534 | |
T | 2.757 (0.977–7.783) | 0.055 | 4.014 (0.952–16.927) | 0.058 | |
M | 1.320 (0.684–2.547) | 0.407 | 0.948 (0.295–3.045) | 0.928 | |
N | 1.908 (0.999–3.645) | 0.050 | 2.916 (0.686–12.400) | 0.147 | |
Risk score | 6.458 (2.708–15.403) | <0.001 | 7.280 (2.500–21.203) | <0.001 |
HR, hazard ratio; CI, confidence interval.
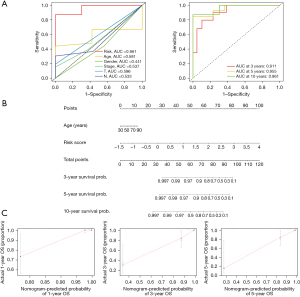
Distribution of the different risk groups and differences in biological pathways
PCA was performed on the low- and high-risk groups, and the results showed that the prognostic risk model could better distinguish the low-risk group and the high-risk groups than the other three groups (Figure 6A), which further supports the accuracy of the signature. In addition, we performed pathway enrichment analysis of the significantly differentially expressed genes between the different risk groups to investigate the potential biological processes affecting the risk score. According to the KEGG results, these differentially expressed genes were mainly enriched in the viral protein interaction with cytokine and cytokine receptor, pantothenate and coenzyme A biosynthesis, and α-linolenic acid metabolism signaling pathways. GO enrichment analysis revealed that these genes were found to be mainly involved in the regulation of macrophage activation, alcohol dehydrogenase (NADP+) activity, and RAGE receptor binding and other related signaling pathways (Figure 6B).
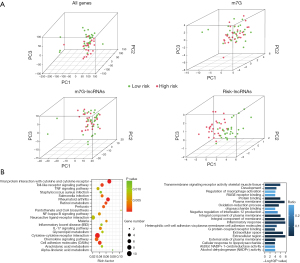
Correlation between the prognostic signature and tumor ICI
Immune cells also play a critical role in the tumor microenvironment. Therefore, it was further investigated whether the risk model associated with the m7G-lncRNA prognostic signature correlates with the expression of 21 tumor-infiltrating immune cell types. The results showed that the infiltration levels of memory B cells (P<0.001), plasma cells (P<0.001), activated memory CD4 T cells (P<0.001), resting natural killer (NK) cells (P<0.001), activated NK cells (P=0.005), monocytes (P=0.006), M0 macrophages (P<0.001), M1 macrophages (P=0.001), M2 macrophages (P<0.001), resting mast cells (P<0.001), and activated mast cells (P<0.001) were significantly different between the low- and high-risk groups (Figure 7A). In addition, the correlations between tumor-infiltrating immune cells in MC tissues (Figure 7B) showed that resting NK cells were negatively correlated with activated NK cells (r=−0.65) and resting mast cells (r=−0.58), respectively. There was also a negative correlation between M0 macrophages and plasma cells (r=−0.52).
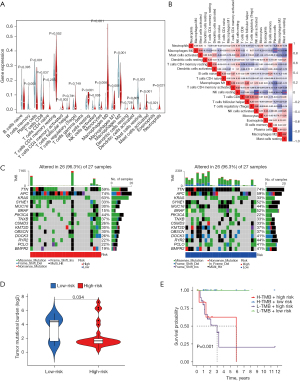
Association of the prognostic signature with TMB
To test the potential value of TMB in MC. TMB analysis was performed on the somatic mutation data of MC of colon cancer obtained from TCGA database. The results showed that TMB was high in all risk groups, reaching 96.3% (Figure 7C). The titin (TTN) gene, the tumor suppressor gene APC, and oncogenes including KRAS and SYNE1 were found to be commonly mutated, but the mutation frequencies of these genes across the different risk groups varied. In high-risk patients, the gene with the highest mutation frequency was APC, and the most common mutation type was multihit. In low-risk patients, the most frequently mutated gene was TTN, and its most frequently mutated type was also multihit. In addition, TMB was significantly different between the risk groups (P=0.034) (Figure 7D). Survival analysis showed that regardless of TMB risk, patients with MC and a high-risk score consistently had poor OS (Figure 7E). These results indicated that TMB may have prognostic significance in patients with MC.
Association of the prognostic signature with IPS
PD1 and CTLA4 were included in the IPS analysis and further divided into four components: ips_ctla4_neg_pd1_neg (negative reaction of CTLA4 and negative reaction of PD1), ips_ctla4_neg_pd1_pos (negative reaction of CTLA4 and positive reaction of PD1), ips_ctla4_pos_pd1_neg (positive reaction of CTLA4 and negative reaction of PD1), and ips_ctla4_pos_pd1_pos (positive reaction of CTLA4 and positive reaction of PD1). In different risk groups, the mean IPS showed no significant differences in the four components of the negative or positive response to PD1 and CTLA4 (Figure S3). These results suggest that this prognostic signature may lack efficacy in risk score models that predict response to treatment with PD1 and CTLA4.
m7G modification prediction results
m7GFinder is a proven high precision predictor based on deep neural network models. Users only need to input the standard FASTA format of the RNA sequence to achieve m7G modification prediction of direct RNA sequencing samples (32). We predicted five lncRNAs that had previously included prognostic signatures (The transcriptome sequence file of AC254629.1 gene could not be found.). The prediction results indicated that m7G modification might occur in LINC01133 and SMIM2-AS1.
Discussion
MC is a relatively common type of colon cancer, with the highest prevalence in people aged 40 to 50 years of age. However, as societal habits change, the incidence of the disease has increased, with younger people tending to be affected. Clinically, early specific symptoms are not obvious, and distant metastases are prone to occur. The disease has a poor prognosis and survival rate and is difficult to cure, thus posing a serious threat to the health of those afflicted (33-35). Surgery remains the first treatment option for this disease, including laparoscopic and open surgery (36). Laparoscopic surgery is widely used due to its high safety, low injury, and short recovery time, but its efficacy is still debated due to limitations in surgical requirements and the level of technology (37). Therefore, it is necessary to explore new prognostic markers for patient diagnosis and prognosis to improve the efficacy of treatment.
RNA methylation, including m5C, m1A, m6A, m7G, etc., is an important epigenetic modification involved in post-transcriptional gene regulation. As one of the most abundant types of methylation modification in RNA, m7G is closely related to the occurrence and development of cancer. Some studies have examined m7G-related lncRNAs as prognostic markers in patients with lung adenocarcinoma (38), gastric cancer (39), pancreatic cancer (40), bladder cancer (41). Taken together, the above results support the use of m7G-related lncRNAs as prognostic and diagnostic markers for a variety of cancers. In this study, we identified and validated six m7G-related lncRNAs (AC254629.1, LINC01133, LINC01134, MHENCR, SMIM2-AS1, and XACT) with prognostic value and established a prognostic signature in MC. To our knowledge, this is the first study to report a predictive assessment of MC-associated lncRNAs linked to m7G-associated genes.
Among the lncRNAs in the constructed prognostic signature, AC254629.1 has been shown to have prognostic value in early CRC (42). LINC01133 has been shown to play an important role in the occurrence and development of gastric (43), epithelial ovarian (44), cervical (45), and pancreatic cancers (46). LINC01134 has been proven to be relevant to immune response and metabolism in hepatocellular carcinoma (HCC) and has also been identified as an effective biomarker for HCC treatment (47,48). Furthermore, MHENCR has been found to be a predictor of poor prognosis in CRC patients and a regulator of tumourigenesis through the inhibition of miR-532-p (49). Vallot et al. demonstrated a unique role for XACT in controlling the initiation of inactivation of the human X-chromosome (50). However, the involvement of m7G-related lncRNAs in MC has not been found. Therefore, we focused on lncRNAs co-expressed with m7G-related genes in MC and used bioinformatics and statistical techniques to create prognostic signature models of MC.
In this study, we identified differentially expressed m7G-related lncRNAs between MC tissues and adjacent tissues and revealed the prognostic value of m7G-related lncRNAs in MC. More significantly, a novel prognostic signature was identified and confirmed based on differential expression of m7G-lncRNA with prognostic value. Using multivariate Cox and risk scoring methods, we constructed an m7G-lncRNA-associated risk model that divided all MC patients into high-risk and low-risk groups with significant OS differences. According to Kaplan-Meier survival analysis, OS was worse in the high-risk subgroup compared to the low-risk subgroup, regardless of clinical characteristics. ROC curve, nomogram, and calibration chart were used to verify the survival prediction accuracy of m7G-associated lncRNA prognostic signature. Compared with traditional indicators such as cancer grade, stage, and age, the risk scores performed better in predicting patient survival. In addition, enrichment analysis was performed on differentially expressed genes in the different risk groups, which were primarily enriched in immune-related viral protein interactions with cytokines and cytokine receptor signaling pathways. We speculate that the lncRNAs in the prognostic signature may influence MC by modulating immune-related pathways.
Tumor ICI refers to the infiltration of immune cells into the tumor. In colon cancer, ICI with a better prognosis is characterized by high plasma cells, dendritic cells, and mast cells, low CD4+ T cell memory, and M0, M1, and M2 macrophages (51). We investigated the immune status of the different risk score groups and found that different levels of ICI differed between them. In the low-risk group, the infiltration levels of multiple cell types, including memory B cells, plasma cells, activated memory CD4 T cells, resting NK cells, activated NK cells, monocytes, M0 macrophages, M1 macrophages, M2 macrophages, resting mast cells, activated mast cells, and neutrophils, were significantly higher than that in the high-risk group. These results suggest that various tumor immune cell characteristics in MC patients can be distinguished based on risk scores of m7G-associated lncRNA prognostic signature. TMB refers to the total number of mutations per megabyte in tumor tissue and has also become a biomarker for immunological testing and prognostic analysis in a variety of cancers (52,53). It is believed that a high TMB state is associated with more tumor neoantigens, and that more tumor neoantigens present on the surface of tumor cells may be recognized by immune cells and activate the body’s immune system to kill tumors. Colon cancer patients with high TMB (TMB ≥8 muts/Mb) have been reported to exhibit longer OS than colon cancer patients with low TMB (54,55). In this study, we also explored the correlation between the prognostic signature and TMB. We found that several classical tumor-related genes, such as APC, TTN, and TP53, also showed a high mutation frequency in the two risk subgroups in the TMB analysis. There were significant differences in TMB across risk groups, with patients in the high-risk and high-TMB groups having the worst survival. The results suggested that the prognostic signature is able to predict the TMB of the patient and that the combination of TMB and prognostic signature may be effective in guiding the prognosis prediction and immunoefficacy of patients.
Despite these promising findings, some limitations to this study should be addressed. First, the experiment was based on the case data from a public database, TCGA, which could have involved bias from the included cases. Second, this study mainly used bioinformatic analysis methods, and the expression of this gene should be further verified by clinical and cell line-specific experiments.
Conclusions
In this study, we screened and constructed six m7G-associated lncRNAs as prognostic signatures based on the clinical and transcriptomic data of TCGA and confirmed its good performance in the prognosis of mucinous colonic adenocarcinoma. Finally, we also evaluated the correlation between the prognostic signature and TMB, IPS, and showed that the combination of TMB and prognostic signature better predicted patients’ survival. In conclusion, m7G-associated lncRNA prognostic signatures are potentially valuable for the prognosis and diagnosis of mucinous colonic adenocarcinoma.
Acknowledgments
Funding: This study was funded by
Footnote
Reporting Checklist: The authors have completed the TRIPOD reporting checklist. Available at https://jgo.amegroups.com/article/view/10.21037/jgo-23-980/rc
Peer Review File: Available at https://jgo.amegroups.com/article/view/10.21037/jgo-23-980/prf
Conflicts of Interest: All authors have completed the ICMJE uniform disclosure form (available at https://jgo.amegroups.com/article/view/10.21037/jgo-23-980/coif). The authors have no conflicts of interest to declare.
Ethical Statement: The authors are accountable for all aspects of the work in ensuring that questions related to the accuracy or integrity of any part of the work are appropriately investigated and resolved. The study was conducted in accordance with the Declaration of Helsinki (as revised in 2013).
Open Access Statement: This is an Open Access article distributed in accordance with the Creative Commons Attribution-NonCommercial-NoDerivs 4.0 International License (CC BY-NC-ND 4.0), which permits the non-commercial replication and distribution of the article with the strict proviso that no changes or edits are made and the original work is properly cited (including links to both the formal publication through the relevant DOI and the license). See: https://creativecommons.org/licenses/by-nc-nd/4.0/.
References
- Sung H, Ferlay J, Siegel RL, et al. Global Cancer Statistics 2020: GLOBOCAN Estimates of Incidence and Mortality Worldwide for 36 Cancers in 185 Countries. CA Cancer J Clin 2021;71:209-49. [Crossref] [PubMed]
- Degro CE, Strozynski R, Loch FN, et al. Survival rates and prognostic factors in right- and left-sided colon cancer stage I-IV: an unselected retrospective single-center trial. Int J Colorectal Dis 2021;36:2683-96. [Crossref] [PubMed]
- Nitsche U, Friess H, Agha A, et al. Prognosis of mucinous and signet-ring cell colorectal cancer in a population-based cohort. J Cancer Res Clin Oncol 2016;142:2357-66. [Crossref] [PubMed]
- Kleihues P, Sobin LH. World Health Organization classification of tumors. Cancer 2000;88:2887. [Crossref] [PubMed]
- Dutta A, Roy A, Chatterjee S. Long noncoding RNAs in cancer immunity: a new avenue in drug discovery. Drug Discov Today 2021;26:264-72. [Crossref] [PubMed]
- Guo CJ, Ma XK, Xing YH, et al. Distinct Processing of lncRNAs Contributes to Non-conserved Functions in Stem Cells. Cell 2020;181:621-636.e22. [Crossref] [PubMed]
- Zuckerman B, Ron M, Mikl M, et al. Gene Architecture and Sequence Composition Underpin Selective Dependency of Nuclear Export of Long RNAs on NXF1 and the TREX Complex. Mol Cell 2020;79:251-267.e6. [Crossref] [PubMed]
- Lalevée S, Feil R. Long noncoding RNAs in human disease: emerging mechanisms and therapeutic strategies. Epigenomics 2015;7:877-9. [Crossref] [PubMed]
- Zhang X, Mao L, Li L, et al. Long noncoding RNA GIHCG functions as an oncogene and serves as a serum diagnostic biomarker for cervical cancer. J Cancer 2019;10:672-81. [Crossref] [PubMed]
- Işın M, Uysaler E, Özgür E, et al. Exosomal lncRNA-p21 levels may help to distinguish prostate cancer from benign disease. Front Genet 2015;6:168. [Crossref] [PubMed]
- Fang D, Ou X, Sun K, et al. m6A modification-mediated lncRNA TP53TG1 inhibits gastric cancer progression by regulating CIP2A stability. Cancer Sci 2022;113:4135-50. [Crossref] [PubMed]
- Boccaletto P, Stefaniak F, Ray A, et al. MODOMICS: a database of RNA modification pathways. 2021 update. Nucleic Acids Res 2022;50:D231-5. [Crossref] [PubMed]
- Malbec L, Zhang T, Chen YS, et al. Dynamic methylome of internal mRNA N(7)-methylguanosine and its regulatory role in translation. Cell Res 2019;29:927-41. [Crossref] [PubMed]
- Luo Y, Yao Y, Wu P, et al. The potential role of N(7)-methylguanosine (m7G) in cancer. J Hematol Oncol 2022;15:63. [Crossref] [PubMed]
- Chen Z, Zhu W, Zhu S, et al. METTL1 promotes hepatocarcinogenesis via m(7) G tRNA modification-dependent translation control. Clin Transl Med 2021;11:e661. [Crossref] [PubMed]
- Chen J, Li K, Chen J, et al. Aberrant translation regulated by METTL1/WDR4-mediated tRNA N7-methylguanosine modification drives head and neck squamous cell carcinoma progression. Cancer Commun (Lond) 2022;42:223-44. [Crossref] [PubMed]
- Ying X, Liu B, Yuan Z, et al. METTL1-m(7) G-EGFR/EFEMP1 axis promotes the bladder cancer development. Clin Transl Med 2021;11:e675. [Crossref] [PubMed]
- Liu Y, Yang C, Zhao Y, et al. Overexpressed methyltransferase-like 1 (METTL1) increased chemosensitivity of colon cancer cells to cisplatin by regulating miR-149-3p/S100A4/p53 axis. Aging (Albany NY) 2019;11:12328-44. [Crossref] [PubMed]
- Liu P, Dong C, Shi H, et al. Constructing and validating of m7G-related genes prognostic signature for hepatocellular carcinoma and immune infiltration: potential biomarkers for predicting the overall survival. J Gastrointest Oncol 2022;13:3169-82. [Crossref] [PubMed]
- He R, Man C, Huang J, et al. Identification of RNA Methylation-Related lncRNAs Signature for Predicting Hot and Cold Tumors and Prognosis in Colon Cancer. Front Genet 2022;13:870945. [Crossref] [PubMed]
- Cheng Z, Wang J, Xu Y, et al. N7-methylguanosine-related lncRNAs: Distinction between hot and cold tumors and construction of predictive models in colon adenocarcinoma. Front Oncol 2022;12:951452. [Crossref] [PubMed]
- Ma X, Yang B, Yang Y, et al. Identification of N(7)-methylguanosine-related IncRNA signature as a potential predictive biomarker for colon adenocarcinoma. Front Genet 2022;13:946845. [Crossref] [PubMed]
- Yang S, Zhou J, Chen Z, et al. A novel m7G-related lncRNA risk model for predicting prognosis and evaluating the tumor immune microenvironment in colon carcinoma. Front Oncol 2022;12:934928. [Crossref] [PubMed]
- Ritchie ME, Phipson B, Wu D, et al. limma powers differential expression analyses for RNA-sequencing and microarray studies. Nucleic Acids Res 2015;43:e47. [Crossref] [PubMed]
- Villanueva RAM, Chen ZJ. ggplot2: Elegant Graphics for Data Analysis (2nd ed.). Measurement: Interdisciplinary Research and Perspectives 2019;17:160-67.
- Brunson JC. ggalluvial: Layered Grammar for Alluvial Plots. J Open Source Softw 2020;5:2017. [Crossref] [PubMed]
- Friedman J, Hastie T, Tibshirani R. Regularization Paths for Generalized Linear Models via Coordinate Descent. J Stat Softw 2010;33:1-22.
- Blanche P, Dartigues JF, Jacqmin-Gadda H. Estimating and comparing time-dependent areas under receiver operating characteristic curves for censored event times with competing risks. Stat Med 2013;32:5381-97. [Crossref] [PubMed]
- Mayakonda A, Lin DC, Assenov Y, et al. Maftools: efficient and comprehensive analysis of somatic variants in cancer. Genome Res 2018;28:1747-56. [Crossref] [PubMed]
- Zhang Y, Jiang J, Ma J, et al. DirectRMDB: a database of post-transcriptional RNA modifications unveiled from direct RNA sequencing technology. Nucleic Acids Res 2023;51:D106-16. [Crossref] [PubMed]
- Song B, Tang Y, Chen K, et al. m7GHub: deciphering the location, regulation and pathogenesis of internal mRNA N7-methylguanosine (m7G) sites in human. Bioinformatics 2020;36:3528-36. [Crossref] [PubMed]
- Wang X, Zhang Y, Chen K, et al. m7GHub V2.0: an updated database for decoding the N7-methylguanosine (m7G) epitranscriptome. Nucleic Acids Res 2024;52:D203-12. [Crossref] [PubMed]
- Lian L, Xu XF, Shen XM, et al. Pattern of distant metastases and predictive nomograms in colorectal mucinous adenocarcinoma: a SEER analysis. J Gastrointest Oncol 2021;12:2906-18. [Crossref] [PubMed]
- Guarini C, Todisco A, Tucci M, et al. Massive hyper-progression during anti-PD-1 immunotherapy in a young patient with metastatic mucinous adenocarcinoma of the right colon: a case report and literature review. Precis Cancer Med 2021;4:30.
- Xu X, Shen W, Wang D, et al. Clinical features and prognosis of resectable pulmonary primary invasive mucinous adenocarcinoma. Transl Lung Cancer Res 2022;11:420-31. [Crossref] [PubMed]
- Matsuda T, Sumi Y, Yamashita K, et al. Optimal Surgery for Mid-Transverse Colon Cancer: Laparoscopic Extended Right Hemicolectomy Versus Laparoscopic Transverse Colectomy. World J Surg 2018;42:3398-404. [Crossref] [PubMed]
- Di Buono G, Buscemi S, Cocorullo G, et al. Feasibility and Safety of Laparoscopic Complete Mesocolic Excision (CME) for Right-sided Colon Cancer: Short-term Outcomes. A Randomized Clinical Study. Ann Surg 2021;274:57-62. [Crossref] [PubMed]
- Zhang C, Zhou D, Wang Z, et al. Risk Model and Immune Signature of m7G-Related lncRNA Based on Lung Adenocarcinoma. Front Genet 2022;13:907754. [Crossref] [PubMed]
- Ma M, Li J, Zeng Z, et al. Integrated analysis from multicentre studies identities m7G-related lncRNA-derived molecular subtypes and risk stratification systems for gastric cancer. Front Immunol 2023;14:1096488. [Crossref] [PubMed]
- Lu J, Yang P, Yu L, et al. Identification of m7G-Related LncRNA Signature for Predicting Prognosis and Evaluating Tumor Immune Infiltration in Pancreatic Adenocarcinoma. Diagnostics (Basel) 2023;13:1697. [Crossref] [PubMed]
- Li Z, Zhao J, Huang X, et al. An m7G-related lncRNA signature predicts prognosis and reveals the immune microenvironment in bladder cancer. Sci Rep 2023;13:4302. [Crossref] [PubMed]
- Xiong Z, Li X, Yin S, et al. Prognostic Value of N6-Methyladenosine-Related lncRNAs in Early-Stage Colorectal Cancer: Association With Immune Cell Infiltration and Chemotherapeutic Drug Sensitivity. Front Mol Biosci 2021;8:724889. [Crossref] [PubMed]
- Yang XZ, Cheng TT, He QJ, et al. LINC01133 as ceRNA inhibits gastric cancer progression by sponging miR-106a-3p to regulate APC expression and the Wnt/β-catenin pathway. Mol Cancer 2018;17:126. [Crossref] [PubMed]
- Liu S, Xi X. LINC01133 contribute to epithelial ovarian cancer metastasis by regulating miR-495-3p/TPD52 axis. Biochem Biophys Res Commun 2020;533:1088-94. [Crossref] [PubMed]
- Feng Y, Qu L, Wang X, et al. LINC01133 promotes the progression of cervical cancer by sponging miR-4784 to up-regulate AHDC1. Cancer Biol Ther 2019;20:1453-61. [Crossref] [PubMed]
- Weng YC, Ma J, Zhang J, et al. Long non-coding RNA LINC01133 silencing exerts antioncogenic effect in pancreatic cancer through the methylation of DKK1 promoter and the activation of Wnt signaling pathway. Cancer Biol Ther 2019;20:368-80. [Crossref] [PubMed]
- Li X, Zhang Z, Liu M, et al. Establishment of a lncRNA-Based Prognostic Gene Signature Associated With Altered Immune Responses in HCC. Front Immunol 2022;13:880288. [Crossref] [PubMed]
- Wang Z, Wang X, Rong Z, et al. LncRNA LINC01134 Contributes to Radioresistance in Hepatocellular Carcinoma by Regulating DNA Damage Response via MAPK Signaling Pathway. Front Pharmacol 2021;12:791889. [Crossref] [PubMed]
- Zhou D, Liao Z, Chen X, et al. LncRNA MHENCR Predicts Poor Outcomes in Patients with Colorectal Carcinoma and Modulates Tumorigenesis by Impairing MiR-532-3p. Tohoku J Exp Med 2022;259:77-84. [Crossref] [PubMed]
- Vallot C, Huret C, Lesecque Y, et al. XACT, a long noncoding transcript coating the active X chromosome in human pluripotent cells. Nat Genet 2013;45:239-41. [Crossref] [PubMed]
- Guo JN, Chen D, Deng SH, et al. Identification and quantification of immune infiltration landscape on therapy and prognosis in left- and right-sided colon cancer. Cancer Immunol Immunother 2022;71:1313-30. [Crossref] [PubMed]
- Lazăr DC, Avram MF, Romoșan I, et al. Prognostic significance of tumor immune microenvironment and immunotherapy: Novel insights and future perspectives in gastric cancer. World J Gastroenterol 2018;24:3583-616. [Crossref] [PubMed]
- Addeo A, Friedlaender A, Banna GL, et al. TMB or not TMB as a biomarker: That is the question. Crit Rev Oncol Hematol 2021;163:103374. [Crossref] [PubMed]
- Chan TA, Yarchoan M, Jaffee E, et al. Development of tumor mutation burden as an immunotherapy biomarker: utility for the oncology clinic. Ann Oncol 2019;30:44-56. [Crossref] [PubMed]
- Innocenti F, Ou FS, Qu X, et al. Mutational Analysis of Patients With Colorectal Cancer in CALGB/SWOG 80405 Identifies New Roles of Microsatellite Instability and Tumor Mutational Burden for Patient Outcome. J Clin Oncol 2019;37:1217-27. [Crossref] [PubMed]